Pass Microsoft Data Science DP-100 Exam in First Attempt Easily
Latest Microsoft Data Science DP-100 Practice Test Questions, Data Science Exam Dumps
Accurate & Verified Answers As Experienced in the Actual Test!
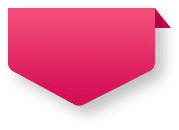
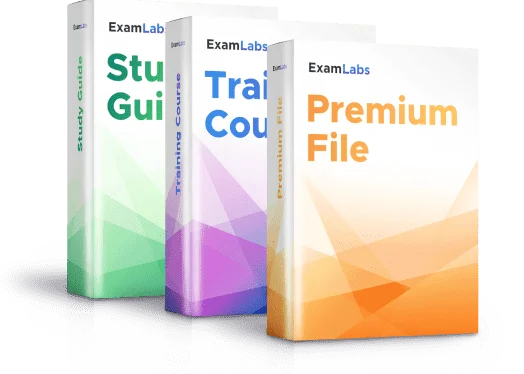
Check our Last Week Results!



- Premium File 517 Questions & Answers
Last Update: Oct 13, 2024 - Training Course 80 Lectures
- Study Guide 608 Pages
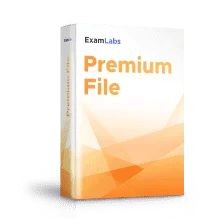
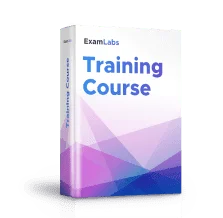
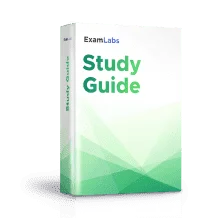
Download Free Microsoft Data Science DP-100 Exam Dumps, Data Science Practice Test
File Name | Size | Downloads | |
---|---|---|---|
microsoft |
4.7 MB | 1090 | Download |
microsoft |
5.1 MB | 1113 | Download |
microsoft |
4.6 MB | 1262 | Download |
microsoft |
3.9 MB | 1145 | Download |
microsoft |
3.8 MB | 1236 | Download |
microsoft |
3.7 MB | 1336 | Download |
microsoft |
4.2 MB | 1456 | Download |
microsoft |
4.4 MB | 1599 | Download |
microsoft |
4.4 MB | 1719 | Download |
microsoft |
3.4 MB | 1575 | Download |
microsoft |
4.7 MB | 1581 | Download |
microsoft |
1.2 MB | 1946 | Download |
microsoft |
1.2 MB | 1914 | Download |
Free VCE files for Microsoft Data Science DP-100 certification practice test questions and answers, exam dumps are uploaded by real users who have taken the exam recently. Download the latest DP-100 Designing and Implementing a Data Science Solution on Azure certification exam practice test questions and answers and sign up for free on Exam-Labs.
Microsoft Data Science DP-100 Practice Test Questions, Microsoft Data Science DP-100 Exam dumps
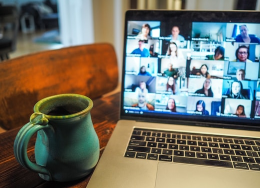
Basics of Machine Learning
1. What You Will Learn in This Section
Hello and welcome to the first section of the Azure machine learning course. In this section, we will cover why machine learning is the future, why data is the new oil, and we will look at some of the greatest examples of machine learning in the real world. We will briefly touch base on why learning Azure ML is so important, along with some fascinating case studies of Hershey's. With an example of how a kid learns and then understanding how the machine learns, many traditional systems use a rule-based algorithm. It will be worthwhile to see how machine learning can help us enhance those systems. We will also cover what we mean by supervised and unsupervised learning. Now, before we start working on any type of model, it is very important to understand what kind of data we are dealing with. Whether it is all numeric or string, whether it is categorical or continuous, what are the dependent or independent variables in the data? Your decision to choose an algorithm is greatly influenced by the data you are dealing with. We’ll also go through some of the common terms such as probability, mean, median, mode and range, and some more. While some of you might be aware of these terms, I thought of including these forth benefit of the larger audience. Finally, I'm going to introduce what are different types of models which are used for solving almost all kinds of machine learning problems. That would include classification regression, what is correlation, how clusters are formed, as well as anomaly detection. So let's get started, and I’ll see you in the next class. Thanks so much for joining me. This one.
2. Why Machine Learning is the Future?
Hello, and welcome to the Azure Machine Learning course. In this lecture, we will see why machine learning is the future. But first, let's try to understand some data measurement. We know that 1 TB is equal to 1000 gigabytes. Many of us have a hard disk.1 petabyte in fact is equal to 1000 terabytes. That’s equivalent to having 1000 hard discs of 1 TB. Going even further, one Exabyte is equal to 1000 petabytes, or Well, if you look at this graph of IDC that gives the prediction of data growth along with the data that has been generated so far, you will be amazed. We have generated 7900 Exabyte’s of data until 2015and if you see the growth pattern we will be generating more than 40,000gb of data by 2020.It is almost impossible to make sense of this amount of data using traditional means of hardware and software. And that is where the machine learning can help us in understanding the data as well as making intelligent business decisions. One more important statistics is that 90% of the entire data in the world has been produced in the last two years alone. So you can imagine at what pace we have been generating the data in the last few years. And it has been done by various industries such as biotech, banking and finance, manufacturing, telecom, ecommerce and of course social media which is the biggest contributor of this. No wonder then that some of the leaders in the industry are calling Big Data and the machine learning which powers it as the next big thing. Horvard Business Review called Data Scientist asthe sexiest job of the 21st century. Also, Microsoft CEO Satin Adela called out machine learning as the key development in his memo to Microsoft in last July. No wonder data is the new oil that weal should be mining as it is going to drive critical business decisions and help companies save Costas well as find out new business avenues. Let’s see how some companies have used this to their advantages. During BR exit, the pound sterling was down by 11%. Just two days after the vote, stock markets across the world crashed. Now this left millions of traders all over the world scrambling to find safer investment positions. However, there was this one company called Omega Point whose customers saw little change in the value of their investments even after the VR exit vote. And the route of investing success during this time by Omega Points customer was a data driven strategy based on machine learning models. They took into account more than 50economic indicators including company specific reports and broader macroeconomic data thus saving millions of dollars for their customers. Another example that all of you must be familiar withies the people you may know ads from LinkedIn. That is also a great example of machine learning. When it was launched, it achieved a click through rate30% higher and generated millions of new page views. And thanks to this, one new feature LinkedIn's growth trajectory shifted significantly upward. Why did it happen? Thanks to the machine learning algorithms running at the background, who would find out? Who are the people who could possibly be associated with this one professional and hence giving him the ad of people you may know? Okay, self-driving cars making product recommendations based on driver identification, Google Home Netflix movie recommendations, and Siri in the Apple iPhone are all very familiar examples of how machine learning is being used in our day-to-day products. So, what are the benefits of machine learning if so many industries are using it? It can be used to pick the best result and help us reach decisions faster. It can also develop insights that are beyond human capabilities and which is based on various patterns derived from the big data. At the same time, it can act at the right time and take advantage of sales opportunities, converting them into closed deals. Which basically means it can have an impact on the revenue as well as the profits of a company. Okay, the next question that you may have is why you should learn Azure ML among so many other products and technologies that are available. Allow me to explain that using Avery fascinating case study of Hershey’s. Hershey’s leveraged the power of machine learning without hiring a data scientist. Unbelievable but true. Hershey’s products are sold in over 63 countries worldwide with a combined revenue of $7.4 billion. And one of the best selling products for Hershey's is drizzlers. At the same time, you must remember that due tithe sheer size of their operations, even a 1% change in the sizing for Twizzlers can result in a savings of half a million dollars for Hershey’s. And consider this as a sugary product. For the products which are based on chocolate, the gains would be much more for a similar gain in percentage. Now, how and why do you think Hershey could achieve this without hiring a data scientist? Well, the answer lies in Azure machine learning capabilities. Let’s look at the capabilities of Azure Machine learning. It’s a complete drag and drop interface and absolutely no programming is required foursome of the basic machine learning models. You can follow a workflow and build a great ML model. It’s as simple as that. Microsoft has also made available large variety of algorithms as modules. All you need to do is simply drag and drop them on your canvas to build your model. And as it requires no coding, deployment, et cetera, you can literally go from experiment to production APIin matter of minutes for simple experiments. Now, this is a very good news for those who knowR and Python as well, because if you have build some models using those languages, you can simply put that code into Azurama and make a model out of that. Azuraml provides a complete flexibility of data storage and this is also one of the key strengths of Azure MLas it supports variety of data storage options. Apart from that, Microsoft also has a large number of pre built APIs available as a service. Now, similar to an app store where you can upload an app and monetize, you can create your prebuilt models and make them available as web services on Microsoft. All right, some of the large companies such as Tata Motors, the makers of Land Rover, Uber, Rolls Royce aircraft engines, Xbox and many, many more have already started using Azure machine learning.Cia.com recently published an article that says Microsoft Azure Machine Learning is a one stopshop for cloud based machine learning. I'm sure by now you are ready to take this step of learning Azure ML and I am equally excited. In the next lecture of what is machine Learning? We will understand the definition of machine learning, how the machines learn, how machine learning is different than the traditional rule based systems, as well as we will look into what is supervised, unsupervised and reinforcement learning. Thank you so much for your time and I'll see you in the next lecture. Until then, have a great time ahead. Bye.
3. What is Machine Learning?
Hello, and welcome to the Azure Machine Learning course. In this lecture, let's try to understand the big question of: What is machine learning? Let's also look into the examples of machine learning, supervised, unsupervised, and reinforcement learning. So what is machine learning? Let's look at the academic and simple definition of machine learning. As per Arthur Samuel, machine learning is the subfield of computer science that gives computers the ability to learn without being explicitly programmed. Now, what does that mean? How does the computer learn? It is very similar to how we humans learn. Human behaviour is usually dependent on the events of the past. Similarly, machine learning algorithms depend heavily on the extraction of knowledge from the past data and build a set of algorithms based on the patterns found in that particular data. Let's try to understand that using how a kid learns. If you show a kid a picture of an apple or hand over an apple and ask, "What is that?" She won't be able to answer because she is unaware of it. However, if we repeatedly tell her that this is an apple and this is an orange, they will be able to identify them correctly after some time. So what has happened here? The brain has actually identified various data points associated with the fruit, such as its color, its shape, its size, et cetera. And it can also identify the differences in the same or among various fruits, but it does not know what it is called. So when we label it as an apple or an orange, the brain assigns the label as the result of those data points. Similarly, when we feed a lot of data to the machine and tell it what it is, the machine learning algorithm then assigns the result to the historic data and can also predict future values of the labels. All right, so why is machine learning important in today's world? Well, traditional It systems depend heavily on the data match or mismatch with the set of direct rules, which then triggers some action. For example, in one of the projects I worked on, there was a rule to audit a refund more than a particular amount. What that meant was every high value tax refund will get audited. Fraudsters are very smart, and they always test the systems to identify such rule based threshold from outside the system. However, a new data analysis driven system that looked at various aspects of a transaction and related entities. It could predict the outcome or the likelihood of an outcome based on historic events and not just direct match or mismatch. Here are some other examples of machine learning a driverless car, a recommendation system that weal most always see while shopping online. Another recent example is that of Uber. Uber is using Microsoft Cognitive Services to offer real time ID. Check. Uber has used Azuramil for identifying who is driving the car by comparing the selfie of the driver with the photographs in their database, thus resulting ina higher security for the passengers and drivers. All right, all of these are great examples of machine learning. Now, machine learning finds its applications across multiple domains such as artificial intelligence, statistics, deep learning and data mining. Okay, so machine learning can be categorized into three categories supervised learning, unsupervised learning and reinforcement learning. Let's take a look at supervised learning with an example. When you were a kid, solutions were provided to you. We all were guided by our parents and guardians. Those solutions and decisions were based on their past experiences. And you learned as you grew. That's nothing but the supervised learning. However, when you start taking your own decisions without any such result or outcome based intelligence, it becomes an unsupervised learning example. This is at a very, very high level, but I hope this is good enough to drive home the point. Let's take a look at it in a bit more technical language. So, in case of supervised learning, the data is labeled, which means both the dependent and the independent variables are provided. Historic data helps us to find out a relationship between X and Y in the form of a function of X. Now, this function can then be used to predict the value of Y if we know the X or the independent variables. Regression analysis, where we try to find out real and continuous value of Y, is an example of supervised learning. We'll look into what we mean by real continuous values and some of these terms we are going to cover when we talk about the basic terms which are being used in the machine learning. Another example could be that of classification such as yes or no or predicting who likes which colour in the data set. Here we have some data that tells us whether the loan of a customer has been approved or not. Such historic data helps us to identify the pattern topredict whether the loan of another customer will be approved or not, provided the values of XR provided. Okay, hope that makes it clear. Let's move on to the unsupervised learning. In case of unsupervised learning, only the X variables are known. The goal of unsupervised learning is to model the underlying structure or distribution in the data in order to learn more about the data. There is no correct answer here and there is no teacher. Algorithms are left on their own to discover and present the interesting structure in the data. Some of the examples that we can give for unsupervised learning are grouping of customers based on their behaviour such as spending patterns such as their preferences, their likes and dislikes, or a recommendation model for products which would belike by a particular set of customers. These are all examples of unsupervised learning. That brings us to the last topic in this lecture, which is reinforcement learning. Let's go back to the example of akid learning in a supervised model and an adult taking some decisions in an unsupervised way. But how do we know if those decisions taken in an unsupervised manner are going to give us the desired result? That is where the reinforcement learning helps us by rewarding the good decisions and penalising the bad ones. It's something like you should pay your bills on time. The reward is a good credit history. Similarly, the unsupervised learning may group a set of customers correctly using their spending patterns as well. And let's say now your marketing department wants to show them a set of advertisements. Now, without any past data, how do we maximise the reward or gain, which in this case is click through rate. This is achieved by reinforcement learning. We would look at these as we deal with some real examples. That concludes this lecture. We covered some basic concepts of what is machine learning, some great examples of how itis being used in the real world and finally the different types of machine learning. In the next class we would look at this mysterious element called data, which seems to have all the answers and is a guiding star of all the machine learning algorithms. So go get yourself a cup of coffee and I will see you in the next class. Until then, have a great time. You.
4. Understanding various aspects of data - Type, Variables, Category
Hello and welcome. In the last lecture we learnt about what is machine learning and some real world examples along with what is supervised, unsupervised and reinforcement learning. We also now under that historic data is crucial for any machine learning algorithms we use, but how to read the data and how to interpret it so that we can make effective use of the same in our algorithm. Remember, garbage in is garbage out. So let's try and understand the data with an example. Now here is a data from a bank that wants to create an algorithm to determine whether customer’s loan should be approved or not. This is the historic data that has been provided to us. Let’s look at what kind of data we are dealing with herein any data set there are three main important factors that we need to understand before we start working on solving the problem using machine learning. Let’s look at the first one. First is the type of variable we are dealing with. So what are we trying to find out here? Well, we need to determine whether the loan application of the customer will be approved or not, or should be approved or not based on the decisions taken by humans in the past, because we want our machine learning algorithm to predict similar results that make status the target or dependent variable, or why in statistical terms. Now let's look at the variables or columns on whom this variable status is dependent. Will loan ID have any impact on these statuses? Well, it's just an ID, some random but unique number. Hence, it is not part of the predictor or independent variable. Gender? Well, we don't know yet. It’s possible, but it won't be the sole criteria. Married may have an impact as some banks give higher points for people who are married and have dependents. Same goes for whether the person is self employed or not as businesses tend to have higher risk compared to a salary person in terms of loan because a fixed salary that gets deposited in the bank at the pay cycle might be valued more in terms of the eligibility for the loan income. Well, no prices for guessing here again how much loan he wants, the credit history and so on. Remember, the credit history here is mentioned as one and zero. That is because it is a derived value and it is possible that banks have decided that they would consider a credit history score above a particular value as good and hence one. Maybe the scores below that is not good and hence zero. I hope you're clear with what is a predictor or independent variable and target or dependent variable. Great. Let's now look at the second important factor, which is nothing but the data type. As you can see from the data set above. Gender, married, self employed, property area and status. They all have character or string as their values, whereas number of dependents income, loan amount, loan term, credit history, they all have numeric values. So at a high level we can safely assume that the data that has been provided to us has these variables as string and these five variables as numeric values. All right, there can be some more type or subtype within them such as integers, float, etc. But as long as we understand these two broad types, we are good. Let's change something here. Now, what would happen to the data type if the bank says that we are going to treat all the customers same if they have three or more dependent on them? In that case, the values above three for number of dependents does not have any impact and we should change it to three plus. In such a scenario it would become a character or a string type of variable. So you should pay very close attention to such details coming from the data provider to avoid any data errors later on in the data processing stage. Okay, so that brings us to the last and very important topic of this lecture which is category of the data. What do we mean by this? Well. If you look at some of the variables here such as gender. Married. Self employed. Credit history. Area and status. They all can have all can have yeFemale. Self-employed or not self-employed status as Y or N. Such variables are known as categorical variables, and in this case, the number of dependents is one of them. Income. Loan amount. Loan term. They can all have a continuous value and any possible range. All right, so variables holding such values are called continuous variables. This is a very important concept and you would apply it when choosing the type of algorithm for predicting the variable. That brings us to the end of this lecture. In this lecture we learned about reading and understanding data using types of variables.
5. Common Machine Learning Terms - Probability, Mean, Mode, Median, Range
Hello and welcome to the third lecture of this section, that is Basics of Machine Learning. In the previous lectures we learned about why machine learningis the future, what is machine learning and we also looked at how to understand the data set. In this lecture we will go through some of the basic terminologies that can be very useful for data processing and data visualization. So let's get started. The first few are pretty easy and straightforward. The first one is mean. Mean is nothing but the average of all values, which is nothing but sum of all observations divided by total number of observations. In this example, we have total eleven observations and the sum is $62,800.Hence the average or mean of this column salary or feature. Salary is five 70 9.9%.Let's look at another term called median. Median is the middle value of the sorted observations with equal number of observations on both sides. What do we mean by that? Let's try to understand this with our sample data. If we plot this data from left to right and in ascending order, you will see that there are equal numbers of observations on both sides of 5800.Hence, 5800 is the median value of this sample set. In case you wonder what the importance of knowing this is, In the case of data cleanup, if some observations have missing values, we can either replace them with mean or median values. We will look at missing values in greater detail in the Data preprocessing section. The next term to understand is mode. Mode is the highest frequency of occurrence of a particular value in the given set of observations. In our sample of observations, 6400 occu All right. Next is a pretty easy and is known as range. Range is nothing but the simple difference between the highest and lowest values in a sample of observations. So in this example, the highest value is 7000 and the lowest is 4000.So our range for the salary column in this data set is 3000.It helps us understand how widely the values are spread in a given set of observations. They all seem pretty easy now, don't they? Follow me now for one of the most important terms that we will cover: probability. We have all heard this term in different ways. One of the most common is that there is a probability that it will rain today. Let's look at what it means in statistical and machine learning terms. As you might have guessed, probability is a numerical way of describing how likely something is going to happen. Well, sample space is a set of possible outcomes that might be observed for an event. If it sounds like Greek to you, let's try and understand that using a simple example of a dice. When we throw a dice, what are the possible outcomes? We can only get one, or two, or three, or four? Five or six—is there any other outcome possible? Well, not unless the dice are faulty. So the sample space for a die is 12345 and 6. So if we throw a die in the air, these are the only possible outcomes. Now, if we want to know what is the probability of three, that is, if I throw a die, what is the likelihood of us getting a three? Well, it is denoted by the letter P, and let's call this event a. So probability of A can be represented as P of A. Okay, so let's go back to what we're talking about, which is the probability of three. So out of six outcomes, how many times do we see the outcome? Three. It only happens once. So the probability of getting a three if we throw a die is one out of six possible outcomes. In numerical terms, the probability of getting a three becomes zero point 16% or 16.67%.Okay, let's take another example. Just to be clear, let's now try to calculate the probability of getting an even number. You may want to pause this video and get the answer. Those are two, four, and six. That is nothing. However, there are three even occurrences out of a total of six occurrences. So the probability of getting an even number is three out of six. That is 0.5% or 50%. Hope this makes sense. Now, this is one of the most important topics, and many machine learning algorithms such as knife bias, logistic algorithms, and so on are based on fundamental principles of probability.
6. Types of Machine Learning Models - Classification, Regression, Clustering etc
Hello and welcome to the last lecture in this section of Basics of Machine Learning. So far we have learned what is machine learning. We also understood how to interpret and understand the data as well as some common terms. In this lecture we are going to cover four fundamental model types that you would build. They are classification, regression, cluster analysis and animal detection. The objective of this lecture is to provide an overview of this model. We will look into the various details of an algorithm and these models when we actually create a model in the respective sections. So let's get started with the first one. All right. The first one we are discussing is classification. Classification is nothing but identifying the categories of the new observation or the record. And how do we do that? We do that on the basis of historic data which has the observation, whose category, membership or classification is known to us. So, based on the historic data, the model will identify the type of category in which the set of records exist. As you can see in this graph, the scattered observations can be grouped into two different classes. And when a new observation is found and plotted on the graph, the model based on the relationship from the previous data can now predict which class of data this new observation belongs to. This type of classification, where we have only two classes or categories, is known as binary or two class classification. However, the set of data can be further divided in multiple classes or groups of data, which then becomes amulet classification as you can see it here’s if you want to create a machine learning model that will identify if the incoming email is a spam or not, you can use a binary or two class classification. However, if the requirement is to further classify anon spam email into primary, social or promotional categories, you will use a multi classification to class. Classifications can also be used if, for example, you want to find out if this customer will default or not, or will a particular customer buy a particular product or availed service. As explained earlier, you can use classification when you are trying to predict the result for a categorical variable. Hope that gives you a brief introduction of classification and where it can be used. The next type is regression, where we try to predict the value of a continuous variable. The primary focus of regression is to find out the relationship between dependent variable and one or more independent variable. In this graph we will have the independent variable in the form of an X and dependent variable as the predicted variable is always a continuous variable such as revenue of the company, price of the product and so on. It is also one of the most common methods used in machine learning. One such graph plotted here suggests that there is a direct correlation between number of medical claims and age of the insured. Now, once such a relationship has been established based on the age value of the data point, the insurer can then predict the number of claims that it may get for any new observation in certain circumstances. It can also be used to infer causal relationship between dependent and independent variable. However, one needs to be a bit careful in drawing a conclusion here, as having a correlation and causal effect are not necessarily the same. For example, people who smoke experience higher level of mental stress. So is smoking the cause of mental stress? Well, not necessarily. Sometimes mental stress may be reason for excessive smoking. So plotting the result on a graph can help us in identifying the type of correlation that exists between two variables. As it can be seen here, when the data points are very close to the regression line and value of increases with X, it suggests that a very strong positive correlation between X and Y exist. This graph shows a weak positive relationship as the points are away from the regression line. This one a strong but negative relationship as the value of Y decreases with increasing while in the last one we can clearly say that there is no linear relationship between X and Hope you are clear on what is regression and where we can use that. Next model is one of my favorites and is called Clustering or cluster analysis. Clustering is the task of grouping a set of objects in such a way that the objects in the same group, we also call it a cluster or observations in the same group are more similar in some sense or another, so they share similar characteristics with each other than the observations in some other groups or some other clusters. It is also worthwhile to note here that it's an unsupervised learning model. One of the examples could be to identify who are those set of customers who are making long-distance calls but don't have a job. Well, most probably they could be students. Clustering is also one of the favorites among marketing people as it provides them the knowledge of a group of customers which they can then use for targeted marketing campaign. All right, that brings us tithe end of cluster analysis. Let’s now briefly touch base on anomaly detection. This one is pretty simple and you can even build this using simple programming languages. However, Aural provides us modules that can detect the animals in the given data set. Anomaly detection is also known as outlier detection and it is the identification of items or events or observations which do not follow an expected pattern or other items in the data set. So typically the animal's items will translate twosome kind of problem such as bank frauds, credit card frauds, structural defects or medical problems. For example, your credit card transaction shows a pattern of usage only in a specific geography. However, if suddenly some random transactions originate which do not conform to the same pattern. Let’s say it's coming from overseas. Then it can trigger an alarm of a possible fraud. One such case I have personally dealt with was that of an income tax assessment. Typically, the income tax assessment in that particular organization used to get completed in a week or two. However, in certain cases it was observed that some assessments were completed in matter of few hours. That was an anomaly which was flagged as such and then investigated with greater success. As we saw, anomalies are also referred to as outliers, novelties noise deviations and exceptions because they do not fit the normal routines or patterns. That brings us to the end of this lecture where we learnt about classification, regression analysis, clustering and anomaly detection. We have now covered a great deal of basics of machine learning and it's time to now understand and start working on Azure ML. I'm so excited to get you started on Azurama in the next class. Until then, thank you so much for joining me in this one and enjoy time.
Microsoft Data Science DP-100 Exam Dumps, Microsoft Data Science DP-100 Practice Test Questions and Answers
Do you have questions about our DP-100 Designing and Implementing a Data Science Solution on Azure practice test questions and answers or any of our products? If you are not clear about our Microsoft Data Science DP-100 exam practice test questions, you can read the FAQ below.
Purchase Microsoft Data Science DP-100 Exam Training Products Individually
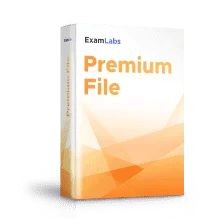
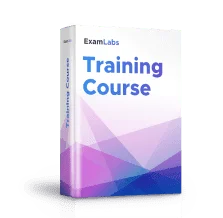
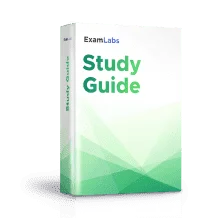
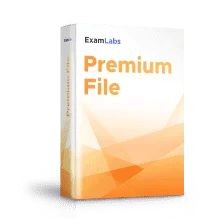
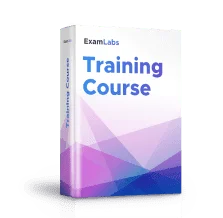
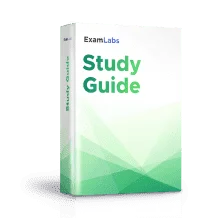